How to Detect Passive and Active Voice in Your Writing Using an LSTM
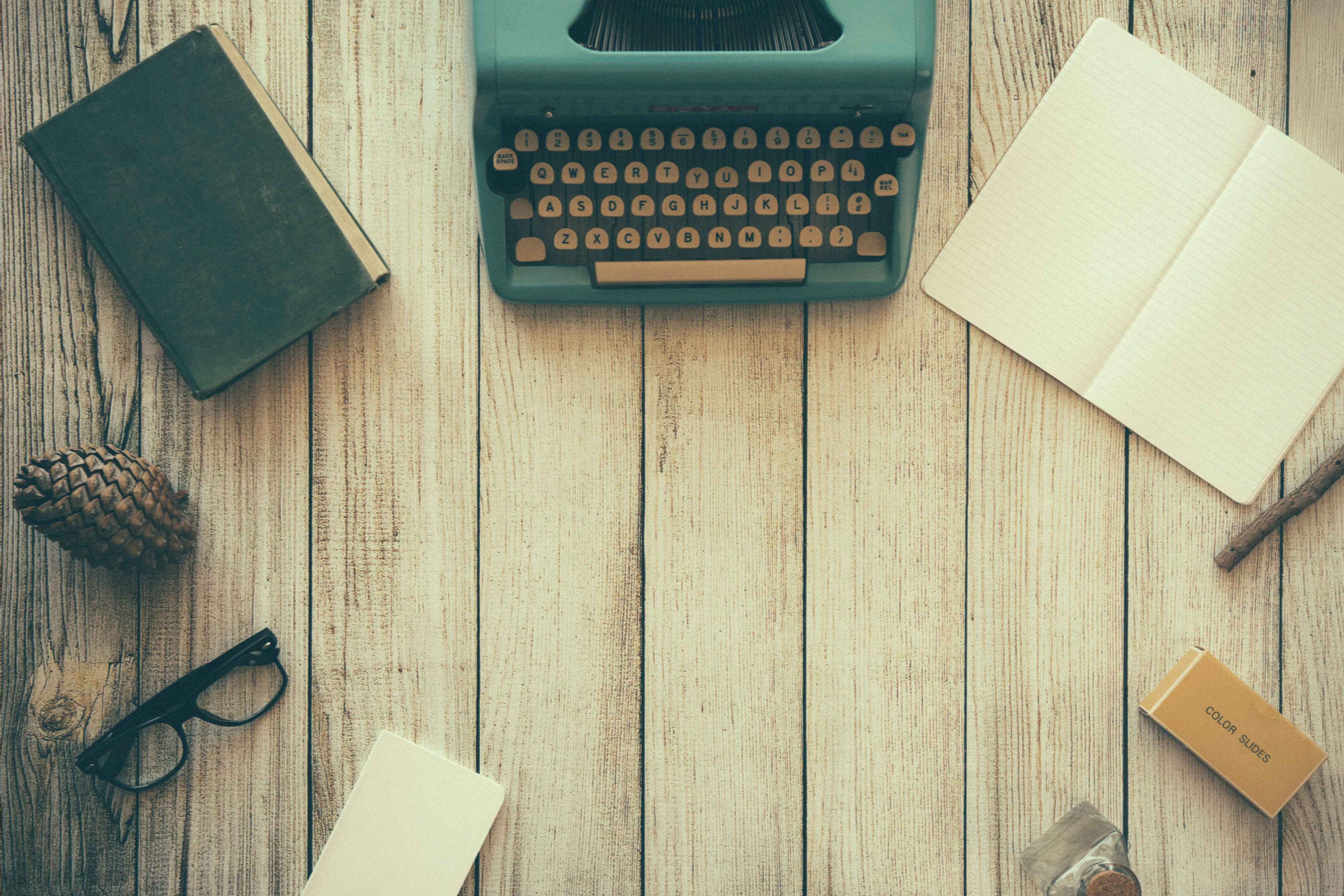
Photo by RetroSupply on Unsplash
Students of humanities and science rejoice! My laziness has proven successful once again. What am I talking about? Well, turns out you can use machine learning to help you become a better writer.
In this post, I show you how you can use a fancy machine learning algorithm to detect that nasty passive voice in your writing.
The Classic style of writing
In the interest of improving my writing, I’ve come across some articles suggesting to use the Classic style.
The Classic style of prose has three tenets:
- Writing is a window onto the world. You have seen something in the world and you want to position the reader so he can see it too with his mind’s eye.
- Write as if you and the reader are equals.
- The goal is to help the reader see objective reality in a conversational style.
A core technique of this kind of writing leverages the active voice. An active voice will have the subject of the sentence performing the action. Whereas a passive voice will have the subject being acted upon.
Writing with an active voice is tantamount to writing as a narrator of ongoing events. This is in contrast to a passive voice where the object being acted upon is made the subject (can you find the passive voice in this sentence?).
Some examples of active voice are:
- “The director will give you instructions.”
- “Water fills a tub.”
The passive voice equivalent to the above examples are:
- “The instruction will be given to you by the director.”
- “A tub is filled by water.”
Ok, so you generally don’t want to use a passive voice in your writing. How might you go about detecting sentences using a passive voice instead of an active one?
A machine learning model to detect passive voice
You could program the rules explicitly. But as with the English language, there will be a multitude of exceptions. There might be a Software 2.0 solution though. Enter long short-term memory (LSTM) recurrent neural networks (RNNs).
I’ll save LSTMs as a topic for another time. For now, just remember that LSTMs are a kind of RNN architecture that tries to address the memory problem of plain RNNs. As such, they work pretty well for machine learning problems around structured text.
I took the following approach to detect passive vs active sentences:
- Collect some examples of passive and active sentences in a CSV file.
- Create an LSTM.
- Test the LSTM on sentences it hasn’t seen.
This is what some of the examples looked like in the CSV file:
Susan will bake two dozen cupcakes for the bake sale,active
The science class viewed the comet,active
The entire stretch of highway was paved by the crew,passive
The novel was read by Mom in one day,passive
I collected around 165 examples with around half active examples and half passive.
I then wrote the code for the LSTM. You can see that below:
"""
Check if a sentence is passive or active.
"""
import torch
from torch import nn
import numpy as np
import pandas as pd
class LSTM(nn.Module):
def __init__(self, input_size, hidden_state_size, num_layers, num_classes, sequence_length):
super(LSTM, self).__init__()
self.hidden_state_size = hidden_state_size
self.sequence_length = hidden_state_size
self.num_layers = num_layers
self.num_classes = num_classes-1
self.sigmoid = nn.Sigmoid()
# Batch_first expects input size (batch_size, sequence_length, input_size)
self.lstm = nn.LSTM(input_size, hidden_state_size, num_layers, batch_first=True)
self.fc1 = nn.Linear(hidden_state_size*sequence_length, self.num_classes)
def forward(self, x):
# x has shape (batch, input_size, sequence_length)
batch_size = x.size(0)
hidden_state = torch.zeros(self.num_layers, batch_size, self.hidden_state_size).to(device) # (num_layers, batch_size, hidden_state)
cell_state = torch.zeros(self.num_layers, batch_size, self.hidden_state_size).to(device)
# Forward prop
output, _ = self.lstm(x, (hidden_state, cell_state)) # (batch_size, sequence_length, hidden_state)
# Reshape but keep batch as first axis and concatenate everything else
# Shape will be: (batch_size*sequence_length, hidden_state_size)
output = output.contiguous().view(batch_size, -1)
output = torch.sigmoid(self.fc1(output))
return output
def one_hot_encode(sequence, dict_size, seq_len, batch_size):
# Creating a multi-dimensional array of zeros with the desired output shape
features = np.zeros((batch_size, seq_len, dict_size), dtype=np.float32)
# Replacing the 0 at the relevant character index with a 1 to represent that character
for i in range(batch_size):
for u in range(seq_len):
features[i, u, sequence[i][u]] = 1
return features
def get_device():
# torch.cuda.is_available() checks and returns a Boolean True if a GPU is available, else it'll return False
is_cuda = torch.cuda.is_available()
# If we have a GPU available, we'll set our device to GPU. We'll use this device variable later in our code.
if is_cuda:
device = torch.device("cuda")
print("GPU is available")
else:
device = torch.device("cpu")
print("GPU not available, CPU used")
return device
def get_prediction(test_sentence):
# Pad
while len(test_sentence) < largest_sentence_length:
test_sentence += ' '
model.eval()
test_sentence = test_sentence.lower()
test_chars = [char for char in test_sentence]
test_chars_sequence = np.array([[char_to_int[char] for char in test_chars]])
encoded_test_chars_sequence = one_hot_encode(test_chars_sequence, input_size, sequence_length, 1)
encoded_test_chars_sequence = torch.from_numpy(encoded_test_chars_sequence).to(device)
# predictions (1 = passive, 0 = active)
output = model(encoded_test_chars_sequence)
return output.item()
def run_test_cases(threshold):
cases = [('Bobby put the cup on the table', 'active'),
('The cup was put on the table by Bobby', 'passive'),
('At dinner, six shrimp were eaten by Harry', 'passive'),
('The house will be cleaned by me every Saturday', 'passive'),
('I will clean the house every Saturday', 'active'),
('The fish was caught by the seagull', 'passive'),
('The dragon has scorched the metropolis with his fiery breath', 'active')]
total = len(cases)
correct = 0
for case in cases:
is_passive = get_prediction(case[0]) > threshold
if is_passive and case[1] == 'passive':
correct+=1
if not is_passive and case[1] == 'active':
correct+=1
# Return accuracy
return correct/total
df = pd.read_csv('data.csv')
data = df.sentence.values
# Find the unique chars and give a unique label to each char
# Create mappings to and from these unique integers
unique_chars = set(''.join(data))
int_to_char = dict(enumerate(unique_chars))
char_to_int = {value: key for key, value in int_to_char.items()}
# Make sure each sentence in the data have the same length (we'll need to pad)
largest_sentence_length = len(max(data, key=len))
# Pad
for i in range(len(data)):
while len(data[i]) < largest_sentence_length:
data[i] += ' '
input_sequence = [each[:-1] for each in data]
target_sequence = df.label.apply(lambda x: 1 if x == 'passive' else 0).values
# Encode the characters
for i in range(len(data)):
input_sequence[i] = [char_to_int[character] for character in input_sequence[i]]
# Hyperparameters
num_layers = 1 # we don't need to stack hidden layers
hidden_state_size = 12
learning_rate = 0.01
num_epochs = 100
num_classes = 2 # space of possibilities for the output
input_size = len(unique_chars) # space of possibilities for the input
sequence_length = largest_sentence_length - 1
batch_size = len(data)
# Encode input (using one hot for simplicity but best to use pre-trained word vectors)
encoded_input_sequence = one_hot_encode(input_sequence, input_size, sequence_length, batch_size)
# Make input and target Torch tensors
device = get_device()
encoded_input_sequence = torch.from_numpy(encoded_input_sequence).to(device) # (batch_size, input_size, sequence_length)
target_sequence = torch.from_numpy(np.array(target_sequence)).to(device)
# Instantiate the model
model = LSTM(input_size, hidden_state_size, num_layers, num_classes, sequence_length).to(device)
print(model)
# Define Loss, Optimizer
loss_function = nn.BCELoss()
optimizer = torch.optim.Adam(model.parameters(), lr=learning_rate)
# Train
for epoch in range(num_epochs):
optimizer.zero_grad() # Clears existing gradients from previous epoch
encoded_input_sequence.to(device)
output = model(encoded_input_sequence)
loss = loss_function(output.view(-1).float(), target_sequence.float())
loss.backward() # Does backpropagation and calculates gradients
optimizer.step() # Updates the weights accordingly
if epoch%10 == 0:
print('Epoch: {}/{}.............'.format(epoch, num_epochs), end=' ')
print("Loss: {:.4f}".format(loss.item()))
print(f'\nAccuracy: {run_test_cases(0.5)}')
Results
Running the code above, you can see the following output (note that your accuracy may be different given the random weight initialization in the neural network):
Epoch: 0/100............. Loss: 0.6896
Epoch: 10/100............. Loss: 0.6948
Epoch: 20/100............. Loss: 0.6178
Epoch: 30/100............. Loss: 0.5244
Epoch: 40/100............. Loss: 0.3662
Epoch: 50/100............. Loss: 0.1889
Epoch: 60/100............. Loss: 0.1849
Epoch: 70/100............. Loss: 0.1238
Epoch: 80/100............. Loss: 0.0766
Epoch: 90/100............. Loss: 0.0492
Accuracy: 0.8571428571428571
An 85% passive voice detection accuracy isn’t too bad given the LSTM was trained on only around 165 examples. As with most neural networks, the more data you have, the better.
But after all these years, I still think it’s cool that you can feed a neural network some examples and it will extract the patterns and rules from them to get to the answer.
And now that I have a machine learning model to watch for passive sentences in my own writing, hopefully the model in my head will start to learn from its feedback too.